12 papers from MIT Jameel Clinic researchers accepted to ICLR 2024
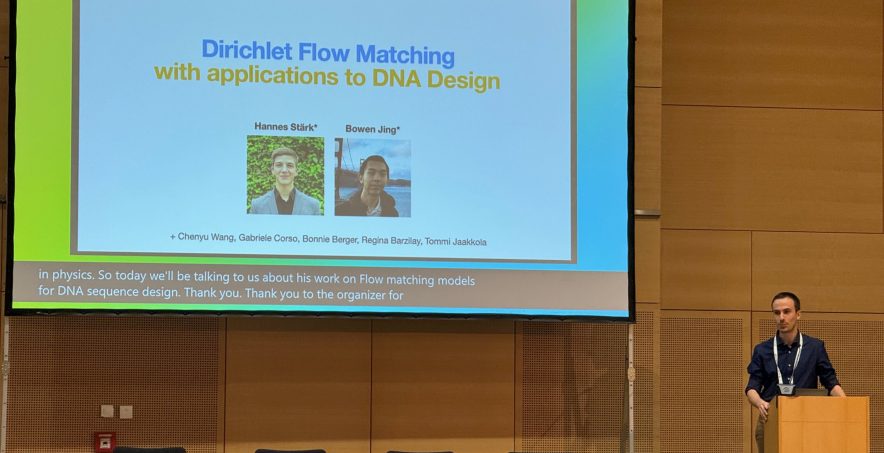
12 papers from the labs of Jameel Clinic Principal Investigators Regina Barzilay, Connor Coley, Marzyeh Ghassemi, Tommi Jaakkola, Dina Katabi, David Sontag, Loza Tadesse, and Caroline Uhler were accepted to the International Conference of Learning Representations (ICLR). Don’t miss out on the two Spotlight papers from the labs of Marzyeh Ghassemi and Dina Katabi.
“Equivariant Scalar Fields for Molecular Docking with Fast Fourier Transforms” by Bowen Jing, Tommi Jaakkola, Bonnie Berger
Researchers developed machine learning methods to speed up the molecular docking process to assist with virtual screening for early drug discovery.
Spotlight: “Views Can Be Deceiving – Improved SSL through Feature Space Augmentation” by Kimia Hamidieh, Haoran Zhang, Swami Sankaranarayanan, Marzyeh Ghassemi
Machine learning models sometimes use “shortcuts,” leading to suboptimal performance for minority subgroups. Research propose a method that developers can use during pretraining to remove spurious features that result in biased performance.
“Conformal Language Modeling” by Victor Quach, Adam Fisch, Tal Schuster, Adam Yala, Jae Ho Sohn, Tommi Jaakkola, Regina Barzilay
Researchers developed an uncertainty estimation and risk control model for generative language models, which can be helpful for generating accurate medical reports.
“Learning Over Molecular Conformer Ensembles: Datasets and Benchmarks” by Yanqiao Zhu, Jeehyun Hwang, Keir Adams, Zhen Liu, Bozhao Nan, Brock Stenfors, Yuanqi Du, Jatin Chauhan, Olaf Wiest, Olexandr Isayev, Connor Coley, Yizhou Sun, Wei Wang
Researchers developed a benchmark called MARCEL to evaluate the potential of learning from a molecular structure dataset and suggest promising research directions.
“Improving protein optimization with smoothed fitness landscapes” by Andrew Kirjner, Jason Yim, Raman Samusevich, Shahar Bracha, Tommi Jaakkola, Regina Barzilay, Ila R Fiete
Researchers developed a model that can predict useful protein mutations to help engineer proteins with useful functions.
“Deep Confident Steps to New Pockets: Strategies for Docking Generalization” by Gabriele Corso, Arthur Deng, Benjamin Fry, Nicholas Polizzi, Regina Barzilay, Tommi Jaakkola
Existing docking models perform poorly tested on proteins that are different from the ones they’re trained on. Researchers developed a model that overcomes these challenges to uncover unknown mechanisms e.g., the toxicity of new drugs or the function of a gene.
“MOFDiff: Coarse-grained Diffusion for Metal-Organic Framework Design” by Xiang Fu, Tian Xie, Andrew Rosen, Tommi Jaakkola, Jake Smith
Metal–organic frameworks (MOFs) are promising in applications such as gas storage and carbon capture. Researchers propose a model that can generate coarse-grained MOF structures, surpassing MOFs in molecular simulations.
Spotlight: “Leveraging Unpaired Data for Vision-Language Generative Models via Cycle Consistency” by Tianhong Li, Sangnie Bhardwaj, Yonglong Tian, Han Zhang, Jarred Barber, Dina Katabi, Guillaume Lajoie, Huiwen Chang, Dilip Krishnan
The automation of image-text correlation is often low quality, but human annotation is costly and time-consuming. Researchers propose an innovative training paradigm that allows vision-language training on unpaired image and text data.
“Removing Biases from Molecular Representations via Information Maximization” by Chenyu Wang, Sharut Gupta, Caroline Uhler, Tommi Jaakkola
Large-scale screening to assess drug effects is challenging due to the high potential for errors. Researchers introduce a framework capable of diverse high-content drug screens.
“Particle Guidance: non-I.I.D. Diverse Sampling with Diffusion Models” by Gabriele Corso,Yilun Xu, Valentin de Bortoli, Regina Barzilay, Tommi Jaakkola
Researchers propose a model that can improve the diversity and sampling efficiency of generative models.
“Learning Inflammatory Biomarkers from Nocturnal Breathing, BMI and Demographics” by Hao He, Dina Katabi
Monitoring inflammation typically requires invasive blood tests. Researchers develop a machine learning model that can detect inflammation and track its progression from nocturnal breathing.
“Sharpness-Aware Minimization (SAM) Improves Classification Accuracy of Bacterial Raman Spectral Data Enabling Portable Diagnostics” by Kaitlin Zareno, Jarett Dewbury, Siamak Sorooshyari, Hossein Mobahi, Loza Tadesse
Researchers propose a machine learning model to assist with portable and rapid disease detection in resource-limited regions and conflict zones.